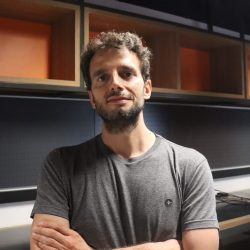
Publicações de João Nuno Barbosa Rodrigues
Lian, Ruixian; Zhou, Dong; Xiao, Lan; Rodrigues, João N. B.; Sheng, Ruilong; Bai, Zhishan; Li, Yulin; Liu, Changsheng PLLA–PEG/mPEG Copolymer with Improved Hydrophilicity, Crystallinity, and Biocompatibility: An In-Depth Study on the Crystallization Kinetics Journal Article Em: ACS Appl. Mater. Interfaces, 2025, ISSN: 1944-8252. Resumo | Links | BibTeX | Tags: Munevar, J.; Silva, K. V. R. A.; Dutra, M. S.; Fabris, F.; Padrón-Hernández, E.; Rodrigues, João N. B.; Cabrera-Baez, M. Chemical pressure effects on the physical properties of the quasicrystal approximants <mml:math xmlns:mml= Journal Article Em: Phys. Rev. B, vol. 111, não 17, 2025, ISSN: 2469-9969. Resumo | Links | BibTeX | Tags: Ogoshi, Elton; Popolin-Neto, Mário; Acosta, Carlos Mera; Nascimento, Gabriel M.; Rodrigues, João N. B.; Oliveira, Osvaldo N.; Paulovich, Fernando V.; Dalpian, Gustavo M. Learning from machine learning: the case of band-gap directness in semiconductors Journal Article Em: Discov Mater, vol. 4, não 1, 2024, ISSN: 2730-7727. Resumo | Links | BibTeX | Tags: General Medicine Ogoshi, Elton; Popolin-Neto, Mário; Acosta, Carlos Mera; Nascimento, Gabriel M.; Rodrigues, João N. B.; Oliveira, Osvaldo N.; Paulovich, Fernando V.; Dalpian, Gustavo M. Learning from machine learning: the case of band-gap directness in semiconductors Journal Article Em: Discov Mater, vol. 4, não 1, 2024, ISSN: 2730-7727. Resumo | Links | BibTeX | Tags: General Medicine Wheeler, William A.; Pathak, Shivesh; Kleiner, Kevin G.; Yuan, Shunyue; Rodrigues, João N. B.; Lorsung, Cooper; Krongchon, Kittithat; Chang, Yueqing; Zhou, Yiqing; Busemeyer, Brian; Williams, Kiel T.; Muñoz, Alexander; Chow, Chun Yu; Wagner, Lucas K. PyQMC: An all-Python real-space quantum Monte Carlo module in PySCF Journal Article Em: vol. 158, não 11, 2023, ISSN: 1089-7690. Resumo | Links | BibTeX | Tags: General Physics and Astronomy, Physical and Theoretical Chemistry Ogoshi, Elton; Ferreira, Henrique; Rodrigues, João N. B.; Dalpian, Gustavo M. Exploring chemical compound space with a graph-based recommender system Working paper 2023. Resumo | Links | BibTeX | Tags: Materials Science2025
@article{Lian2025,
title = {PLLA–PEG/mPEG Copolymer with Improved Hydrophilicity, Crystallinity, and Biocompatibility: An In-Depth Study on the Crystallization Kinetics},
author = {Ruixian Lian and Dong Zhou and Lan Xiao and João N. B. Rodrigues and Ruilong Sheng and Zhishan Bai and Yulin Li and Changsheng Liu},
doi = {10.1021/acsami.5c02818},
issn = {1944-8252},
year = {2025},
date = {2025-05-11},
urldate = {2025-05-11},
journal = {ACS Appl. Mater. Interfaces},
publisher = {American Chemical Society (ACS)},
abstract = {Poly(lactic acid) (PLA) possesses excellent biocompatibility and biodegradability for the construction of biomaterials. However, its limited crystallinity largely restricts practical application. In this study, four poly(l-lactic acid) (PLLA) copolymers were synthesized by incorporating two different molecular weights of PEG/mPEG (1K and 2K) chains with l-lactide via ring-opening polymerization (ROP). The impact of PEG/mPEG chains on the hydrophilicity and mechanical properties of the resulting copolymers, their crystallization kinetics, and activation energy was also examined. The results demonstrate that introducing PEG/mPEG chains could significantly improve the hydrophilicity, crystallinity, and crystallization rate and reduce the maximum crystallization temperature of the copolymer materials. Among the PLLA copolymers, PLLA-mPEG (2K) exhibits the most remarkable improvements in polymer crystallinity, crystallization rate, and reduction of the maximum crystallization temperature. The advancements in copolymer material properties were anticipated to significantly expand the potential applications for PLLA-based polymer materials, which also greatly improved the applicability as sustainable and controllable biopolymer materials.},
keywords = {},
pubstate = {published},
tppubtype = {article}
}
@article{Munevar2025,
title = {Chemical pressure effects on the physical properties of the quasicrystal approximants
doi = {10.1103/physrevb.111.174408},
issn = {2469-9969},
year = {2025},
date = {2025-05-06},
urldate = {2025-05-00},
journal = {Phys. Rev. B},
volume = {111},
number = {17},
publisher = {American Physical Society (APS)},
abstract = {Quasicrystal approximants (QCAs) are a class of crystalline materials that share structural and electronic similarities with quasicrystals while maintaining periodicity. Here we report on the structural, thermodynamic, electronic, magnetic, and transport properties of the QCAs of GdCd6−𝑥Zn𝑥 (𝑥 =0.0, 0.5, and 1.0) prepared by the flux method. The substitution of Cd by Zn results in a 4.7% reduction in volume for 𝑥 =1.0, as revealed by x-ray diffraction measurements. The chemical pressure also reduces the Néel temperature (𝑇𝑁), as observed in specific heat, resistivity, and magnetic susceptibility measurements, which is caused by the reduction of the total density of states at the Fermi level induced by Zn doping, as suggested from density functional theory calculations. Interestingly, for a specific concentration of Zn (𝑥 = 1.0), the system behaves differently, exhibiting tunable resistance at low temperatures under different values of an external magnetic field. These observations suggest that the physical properties of the GdCd6 approximant under chemical pressure can be interpreted within an electronic multiband scenario.},
keywords = {},
pubstate = {published},
tppubtype = {article}
}
2024
@article{Ogoshi2024,
title = {Learning from machine learning: the case of band-gap directness in semiconductors},
author = {Elton Ogoshi and Mário Popolin-Neto and Carlos Mera Acosta and Gabriel M. Nascimento and João N. B. Rodrigues and Osvaldo N. Oliveira and Fernando V. Paulovich and Gustavo M. Dalpian},
url = {https://link.springer.com/article/10.1007/s43939-024-00073-x},
doi = {10.1007/s43939-024-00073-x},
issn = {2730-7727},
year = {2024},
date = {2024-02-29},
urldate = {2024-12-00},
journal = {Discov Mater},
volume = {4},
number = {1},
publisher = {Springer Science and Business Media LLC},
abstract = {
keywords = {General Medicine},
pubstate = {published},
tppubtype = {article}
}
@article{Ogoshi2024b,
title = {Learning from machine learning: the case of band-gap directness in semiconductors},
author = {Elton Ogoshi and Mário Popolin-Neto and Carlos Mera Acosta and Gabriel M. Nascimento and João N. B. Rodrigues and Osvaldo N. Oliveira and Fernando V. Paulovich and Gustavo M. Dalpian},
url = {https://repositorio.usp.br/directbitstream/1ee44f9f-13de-45ad-bb4b-fb7f0cb95272/3183025%20falta%20rep.pdf},
doi = {10.1007/s43939-024-00073-x},
issn = {2730-7727},
year = {2024},
date = {2024-02-29},
urldate = {2024-12-00},
journal = {Discov Mater},
volume = {4},
number = {1},
publisher = {Springer Science and Business Media LLC},
abstract = {
keywords = {General Medicine},
pubstate = {published},
tppubtype = {article}
}
2023
@article{Wheeler2023,
title = {PyQMC: An all-Python real-space quantum Monte Carlo module in PySCF},
author = {William A. Wheeler and Shivesh Pathak and Kevin G. Kleiner and Shunyue Yuan and João N. B. Rodrigues and Cooper Lorsung and Kittithat Krongchon and Yueqing Chang and Yiqing Zhou and Brian Busemeyer and Kiel T. Williams and Alexander Muñoz and Chun Yu Chow and Lucas K. Wagner},
doi = {10.1063/5.0139024},
issn = {1089-7690},
year = {2023},
date = {2023-03-21},
volume = {158},
number = {11},
publisher = {AIP Publishing},
abstract = {
keywords = {General Physics and Astronomy, Physical and Theoretical Chemistry},
pubstate = {published},
tppubtype = {article}
}
@workingpaper{ogoshi2023exploring,
title = {Exploring chemical compound space with a graph-based recommender system},
author = {Elton Ogoshi and Henrique Ferreira and João N. B. Rodrigues and Gustavo M. Dalpian},
url = {https://arxiv.org/abs/2306.16496},
doi = {10.48550/arXiv.2306.16496},
year = {2023},
date = {2023-01-01},
urldate = {2023-01-01},
abstract = {With the availability of extensive databases of inorganic materials, data-driven approaches leveraging machine learning have gained prominence in materials science research. In this study, we propose an innovative adaptation of data-driven concepts to the mapping and exploration of chemical compound space. Recommender systems, widely utilized for suggesting items to users, employ techniques such as collaborative filtering, which rely on bipartite graphs composed of users, items, and their interactions. Building upon the Open Quantum Materials Database (OQMD), we constructed a bipartite graph where elements from the periodic table and sites within crystal structures are treated as separate entities. The relationships between them, defined by the presence of ions at specific sites and weighted according to the thermodynamic stability of the respective compounds, allowed us to generate an embedding space that contains vector representations for each ion and each site. Through the correlation of ion-site occupancy with their respective distances within the embedding space, we explored new ion-site occupancies, facilitating the discovery of novel stable compounds. Moreover, the graph's embedding space enabled a comprehensive examination of chemical similarities among elements, and a detailed analysis of local geometries of sites. To demonstrate the effectiveness and robustness of our method, we conducted a historical evaluation using different versions of the OQMD and recommended new compounds with Kagome lattices, showcasing the applicability of our approach to practical materials design.},
keywords = {Materials Science},
pubstate = {published},
tppubtype = {workingpaper}
}
Orientados e Supervisionados por João Nuno Barbosa Rodrigues
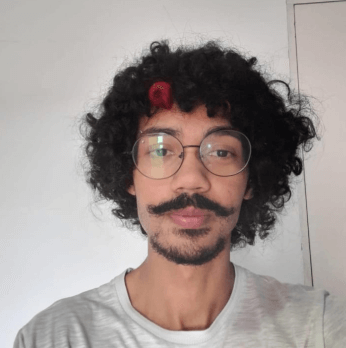
Victor Olimpio
Vínculo: Iniciação Científica
Instituição: Universidade Federal do ABC (UFABC)
Projeto: Estudo de propriedades estruturais, térmicas e eletrônicas de uma liga semicondutora do tipo diamante. (PIC)
Gedeone Bezerra de Almeida Júnior
Vínculo: Iniciação Científica
Instituição: Universidade Federal do Pernambuco (UFPE)
Projeto: Correlações eletrónicas, topologia e magnetismo em duas dimensões
Vínculo: Iniciação Científica
Instituição: Universidade Federal do ABC (UFABC)
Projeto:O problema do bandgap direto e indireto — um estudo ab initio em sistemas um-dimensionais