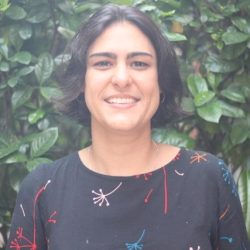
Publicações de Luana Pedroza
Gomes-Filho, Márcio S.; Torres, Alberto; Rocha, Alexandre Reily; Pedroza, Luana S. Size and Quality of Quantum Mechanical Data Set for Training Neural Network Force Fields for Liquid Water Journal Article Em: The Journal of Physical Chemistry B, vol. 127, não 6, pp. 1422-1428, 2023, (PMID: 36730848). Resumo | Links | BibTeX | Tags: 2023
@article{doi:10.1021/acs.jpcb.2c09059,
title = {Size and Quality of Quantum Mechanical Data Set for Training Neural Network Force Fields for Liquid Water},
author = {Márcio S. Gomes-Filho and Alberto Torres and Alexandre Reily Rocha and Luana S. Pedroza},
url = {https://doi.org/10.1021/acs.jpcb.2c09059},
doi = {10.1021/acs.jpcb.2c09059},
year = {2023},
date = {2023-01-01},
journal = {The Journal of Physical Chemistry B},
volume = {127},
number = {6},
pages = {1422-1428},
abstract = {Molecular dynamics simulations have been used in different scientific fields to investigate a broad range of physical systems. However, the accuracy of calculation is based on the model considered to describe the atomic interactions. In particular, ab initio molecular dynamics (AIMD) has the accuracy of density functional theory (DFT) and thus is limited to small systems and a relatively short simulation time. In this scenario, Neural Network Force Fields (NNFFs) have an important role, since they provide a way to circumvent these caveats. In this work, we investigate NNFFs designed at the level of DFT to describe liquid water, focusing on the size and quality of the training data set considered. We show that structural properties are less dependent on the size of the training data set compared to dynamical ones (such as the diffusion coefficient), and a good sampling (selecting data reference for the training process) can lead to a small sample with good precision.},
note = {PMID: 36730848},
keywords = {},
pubstate = {published},
tppubtype = {article}
}
Orientados e Supervisionados por Luana Pedroza
João Rheinheimer
Vínculo: Mestrado
Instituição: Universidade Federal do ABC (UFABC)
Projeto: Efeitos nucleares quânticos para moléculas absorvidas em superfícies metálicas. (FAPESP)