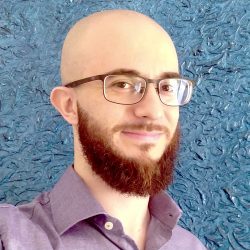
Publicações de Daniel Cassar
Silva, Roni A.; Batista, Gislene; Bradtmüller, Henrik; Campos, João V.; Martins, Gabriela K.; Cassar, Daniel R.; Kurelo, Bruna C. E. S.; Zallocco, Vinicius M.; Rodrigues, Ana C. M.; Cassanjes, Fabia C.; Poirier, Gael Y.; Serbena, Francisco C. Structure–property relationships in sodium phosphate glasses and glass‐ceramics containing tantalum oxide Journal Article Em: J Am Ceram Soc., 2025, ISSN: 1551-2916. Resumo | Links | BibTeX | Tags: Stoco, Caroline Binde; Cassar, Daniel R.; Santana, Geovana Lira; Kaufman, Michael; Clarke, Amy; Coury, Francisco Gil Optimizing toughness in high entropy alloys using a genetic algorithm: A combined computational and experimental approach Journal Article Em: Materials Today Communications, vol. 41, 2024, ISSN: 2352-4928. Resumo | Links | BibTeX | Tags: Acosta, María Helena Ramírez; Cassar, Daniel R.; Rodrigues, Lorena Raphael; Baldin, João Marcos Conradi; Zanotto, Edgar Dutra Diffusion proxies reveal the dynamic process in supercooled and glassy lithium diborate Journal Article Em: Ceramics International, 2024, ISSN: 0272-8842. Resumo | Links | BibTeX | Tags: Classical nucleation theory, Crystal nucleation, Diffusion coefficient, Lithium diborate Allec, Sarah I.; Lu, Xiaonan; Cassar, Daniel R.; Nguyen, Xuan T.; Hegde, Vinay I.; Mahadevan, Thiruvillamalai; Peterson, Miroslava; Du, Jincheng; Riley, Brian J.; Vienna, John D.; Saal, James E. Evaluation of GlassNet for physics-informed machine learning of glass stability and glass-forming ability Working paper 2024. Resumo | Links | BibTeX | Tags: Silva, Roni Alisson; Batista, Gislene; Cassani, Rodrigo; Teófilo, Ana Flávia; Martins, Gabriela Kobelnik; Cassar, Daniel R.; Serbena, Francisco Carlos; Cassanjes, Fábia; Poirier, Gael Thermal, chemical, and mechanical properties of niobium phosphate glasses and glass-ceramics Journal Article Em: Ceramics International, 2024, ISSN: 0272-8842. Resumo | Links | BibTeX | Tags: Elastic modulus, Glass, Glass-ceramic, Hardness, Niobium, Phosphate Vitoria, Leonardo Santos; Cassar, Daniel R.; Lalic, Susana Souza; Nascimento, Marcio Luis Ferreira Using a simple radial basis function neural network to predict the glass transition temperature of alkali borate glasses Journal Article Em: Journal of Non-Crystalline Solids, vol. 629, pp. 122870, 2024, ISSN: 0022-3093. Resumo | Links | BibTeX | Tags: Artificial neural network, Glass transition temperature, Radial basis function, Simple neural network Cassar, Daniel R. GlassNet: A multitask deep neural network for predicting many glass properties Journal Article Em: Ceramics International, 2023. Welch, Rebecca S.; Zanotto, Edgar D.; Wilkinson, Collin J.; Cassar, Daniel R.; Montazerian, Maziar; Mauro, John C. Cracking the Kauzmann paradox Journal Article Em: Acta Materialia, vol. 254, pp. 118994, 2023, ISSN: 1359-6454. Resumo | Links | BibTeX | Tags: Mannan, Sajid; Zaki, Mohd; Bishnoi, Suresh; Cassar, Daniel R.; Jiusti, Jeanini; Faria, Julio Cesar Ferreira; Christensen, Johan F. S.; Gosvami, Nitya Nand; Smedskjaer, Morten M.; Zanotto, Edgar Dutra; Krishnan, N. M. Anoop Glass hardness: Predicting composition and load effects via symbolic reasoning-informed machine learning Journal Article Em: Acta Materialia, vol. 255, pp. 119046, 2023, ISSN: 1359-6454. Resumo | Links | BibTeX | Tags: Glass composition, Hardness, Indentation load, Indentation Size Effect (ISE), Machine Learning (ML)2025
@article{Silva2025,
title = {Structure–property relationships in sodium phosphate glasses and glass‐ceramics containing tantalum oxide},
author = {Roni A. Silva and Gislene Batista and Henrik Bradtmüller and João V. Campos and Gabriela K. Martins and Daniel R. Cassar and Bruna C. E. S. Kurelo and Vinicius M. Zallocco and Ana C. M. Rodrigues and Fabia C. Cassanjes and Gael Y. Poirier and Francisco C. Serbena},
doi = {10.1111/jace.20677},
issn = {1551-2916},
year = {2025},
date = {2025-05-20},
urldate = {2025-05-20},
journal = {J Am Ceram Soc.},
publisher = {Wiley},
abstract = {
keywords = {},
pubstate = {published},
tppubtype = {article}
}
2024
@article{Stoco2024,
title = {Optimizing toughness in high entropy alloys using a genetic algorithm: A combined computational and experimental approach},
author = {Caroline Binde Stoco and Daniel R. Cassar and Geovana Lira Santana and Michael Kaufman and Amy Clarke and Francisco Gil Coury},
doi = {10.1016/j.mtcomm.2024.110768},
issn = {2352-4928},
year = {2024},
date = {2024-12-00},
urldate = {2024-12-00},
journal = {Materials Today Communications},
volume = {41},
publisher = {Elsevier BV},
abstract = {A genetic algorithm (GA) was developed to search for high entropy alloys (HEAs) with good combinations of mechanical properties. The algorithm was designed find single-phase face-centered cubic (FCC) HEAs, already known for their ductility, with high Hall-Petch constants (K) and high critical resolved shear stresses (
). The objective was to develop a methodology that allows the design of HEAs in a multi-objective environment, focusing on alloys that exhibit enhanced ductility and strength, achieved through an increase in its yield point without significant loss of its ultimate deformation via adjustments of K and
values, resulting in an alloy with high toughness. The most promising alloy suggested by the genetic algorithm was an unconventional composition (Co32.73Cu15.11Fe0.72Hf0.72Mn35.97Mo3.96Ni10.43Sn0.36), with eight different elements in non-equiatomic ratios with maximized K and
values. This alloy was then experimentally produced and characterized by scanning electron microscopy (SEM), synchrotron x-ray diffraction (XRD), transmission electron microscopy (TEM) and microhardness, with the objective of validating the predictions made by the GA. An advantage of the proposed method is the possibility of more systematically identifying and exploring new compositions in the complex composition space characteristic of these multicomponent alloys, as it directs its search towards domains that can be used to solve challenging problems involving multi-objective optimization. However, the thermodynamic parameters used for single-phase FCC prediction, namely the valence electron concentration (VEC) and the dimensionless thermodynamic parameter φ, exhibited limitations. These limitations were further explored in the present study, as evidenced by the fact that the microstructure of the selected alloy was not single-phase, which hindered the study of the mechanical properties, predicted exclusively for monophasic FCC alloys. Therefore, this work highlights the necessity for the development of novel thermodynamic equations for phase prediction, or even the integration of the genetic algorithm with other methodologies, such as CALPHAD calculations, to achieve enhanced results.},
keywords = {},
pubstate = {published},
tppubtype = {article}
}
). The objective was to develop a methodology that allows the design of HEAs in a multi-objective environment, focusing on alloys that exhibit enhanced ductility and strength, achieved through an increase in its yield point without significant loss of its ultimate deformation via adjustments of K and
values, resulting in an alloy with high toughness. The most promising alloy suggested by the genetic algorithm was an unconventional composition (Co32.73Cu15.11Fe0.72Hf0.72Mn35.97Mo3.96Ni10.43Sn0.36), with eight different elements in non-equiatomic ratios with maximized K and
values. This alloy was then experimentally produced and characterized by scanning electron microscopy (SEM), synchrotron x-ray diffraction (XRD), transmission electron microscopy (TEM) and microhardness, with the objective of validating the predictions made by the GA. An advantage of the proposed method is the possibility of more systematically identifying and exploring new compositions in the complex composition space characteristic of these multicomponent alloys, as it directs its search towards domains that can be used to solve challenging problems involving multi-objective optimization. However, the thermodynamic parameters used for single-phase FCC prediction, namely the valence electron concentration (VEC) and the dimensionless thermodynamic parameter φ, exhibited limitations. These limitations were further explored in the present study, as evidenced by the fact that the microstructure of the selected alloy was not single-phase, which hindered the study of the mechanical properties, predicted exclusively for monophasic FCC alloys. Therefore, this work highlights the necessity for the development of novel thermodynamic equations for phase prediction, or even the integration of the genetic algorithm with other methodologies, such as CALPHAD calculations, to achieve enhanced results.@article{RAMIREZACOSTA2024,
title = {Diffusion proxies reveal the dynamic process in supercooled and glassy lithium diborate},
author = {María Helena Ramírez Acosta and Daniel R. Cassar and Lorena Raphael Rodrigues and João Marcos Conradi Baldin and Edgar Dutra Zanotto},
url = {https://www.sciencedirect.com/science/article/pii/S0272884224028153},
doi = {https://doi.org/10.1016/j.ceramint.2024.06.369},
issn = {0272-8842},
year = {2024},
date = {2024-06-27},
urldate = {2024-01-01},
journal = {Ceramics International},
abstract = {Understanding the effective diffusion coefficient (D) is crucial for describing atomic transport during crystal nucleation in supercooled liquids and glasses. However, pinpointing the key structural units driving crystallization in intricate, multicomponent glass-formers remains a challenge. This study presents a novel analysis of lithium diborate (Li₂O·2 B₂O₃ - LB₂) crystallization in supercooled and glassy states by using available viscosity and crystallization data for samples of the same batch. An original key feature is that the nucleation rates were measured using a single-stage rather than the traditional double-stage heat treatment. We compared three proxies for D: Dη obtained from viscosity, DU derived from crystal growth rates, and Dτ estimated from nucleation time-lags. Our analysis revealed that at deep supercoolings, below the glass transition temperature, DU and Dτ yield similar steady-state nucleation rate estimates, which significantly exceed those predicted by using Dη (the most frequently used diffusion proxy). This result suggests that the atomic jumps governing crystal nucleation may be comparable to those in crystal growth, but distinct from those associated with cooperative rearrangements controlling viscous flow. Additionally, the estimated kinetic spinodal temperature (Tks) is above the Kauzmann temperature Tk, implying that crystal nucleation precedes relaxation to the supercooled liquid state, circumventing the entropy paradox and corroborating recent MD simulations for other substances. These findings are valuable for refining theoretical models of crystal nucleation and highlight the complexities of the glassy state.},
keywords = {Classical nucleation theory, Crystal nucleation, Diffusion coefficient, Lithium diborate},
pubstate = {published},
tppubtype = {article}
}
@workingpaper{allec2024evaluation,
title = {Evaluation of GlassNet for physics-informed machine learning of glass stability and glass-forming ability},
author = {Sarah I. Allec and Xiaonan Lu and Daniel R. Cassar and Xuan T. Nguyen and Vinay I. Hegde and Thiruvillamalai Mahadevan and Miroslava Peterson and Jincheng Du and Brian J. Riley and John D. Vienna and James E. Saal},
url = {https://arxiv.org/abs/2403.10682},
doi = {10.48550/arXiv.2403.10682},
year = {2024},
date = {2024-03-19},
urldate = {2024-01-01},
abstract = {Glasses form the basis of many modern applications and also hold great potential for future medical and environmental applications. However, their structural complexity and large composition space make design and optimization challenging for certain applications. Of particular importance for glass processing is an estimate of a given composition's glass-forming ability (GFA). However, there remain many open questions regarding the physical mechanisms of glass formation, especially in oxide glasses. It is apparent that a proxy for GFA would be highly useful in glass processing and design, but identifying such a surrogate property has proven itself to be difficult. Here, we explore the application of an open-source pre-trained NN model, GlassNet, that can predict the characteristic temperatures necessary to compute glass stability (GS) and assess the feasibility of using these physics-informed ML (PIML)-predicted GS parameters to estimate GFA. In doing so, we track the uncertainties at each step of the computation - from the original ML prediction errors, to the compounding of errors during GS estimation, and finally to the final estimation of GFA. While GlassNet exhibits reasonable accuracy on all individual properties, we observe a large compounding of error in the combination of these individual predictions for the prediction of GS, finding that random forest models offer similar accuracy to GlassNet. We also breakdown the ML performance on different glass families and find that the error in GS prediction is correlated with the error in crystallization peak temperature prediction. Lastly, we utilize this finding to assess the relationship between top-performing GS parameters and GFA for two ternary glass systems: sodium borosilicate and sodium iron phosphate glasses. We conclude that to obtain true ML predictive capability of GFA, significantly more data needs to be collected.},
keywords = {},
pubstate = {published},
tppubtype = {workingpaper}
}
@article{SILVA2024,
title = {Thermal, chemical, and mechanical properties of niobium phosphate glasses and glass-ceramics},
author = {Roni Alisson Silva and Gislene Batista and Rodrigo Cassani and Ana Flávia Teófilo and Gabriela Kobelnik Martins and Daniel R. Cassar and Francisco Carlos Serbena and Fábia Cassanjes and Gael Poirier},
url = {https://www.sciencedirect.com/science/article/pii/S0272884224008733},
doi = {https://doi.org/10.1016/j.ceramint.2024.02.350},
issn = {0272-8842},
year = {2024},
date = {2024-02-29},
urldate = {2024-01-01},
journal = {Ceramics International},
abstract = {In this work, glass samples were obtained by melt-quenching in the binary system (100-x)KPO3-xNb2O5 with x = 20, 30, 40, and 50 mol%. Thermal properties investigated by DSC together with structural investigations by Raman spectroscopy allowed to understand the structural effect of Nb2O5 incorporation in the potassium phosphate glass network. A transparent glass-ceramic has also been produced by heat treatment of the 50KPO3–50Nb2O5 glass sample. Density, molar volume, atomic packing, and refractive index were determined and increased with Nb2O5 content. Chemical resistance in several corrosive aqueous solutions was found to increase with Nb2O5 content and crystallization. Vickers hardness as well as elastic modulus and hardness obtained from nanoindentation experiments also strongly increased with higher niobium contents, indicating that not only optical properties but also chemical and mechanical properties are improved with niobium incorporation in the phosphate glass network.},
keywords = {Elastic modulus, Glass, Glass-ceramic, Hardness, Niobium, Phosphate},
pubstate = {published},
tppubtype = {article}
}
@article{VITORIA2024122870,
title = {Using a simple radial basis function neural network to predict the glass transition temperature of alkali borate glasses},
author = {Leonardo Santos Vitoria and Daniel R. Cassar and Susana Souza Lalic and Marcio Luis Ferreira Nascimento},
url = {https://www.sciencedirect.com/science/article/pii/S0022309324000516},
doi = {https://doi.org/10.1016/j.jnoncrysol.2024.122870},
issn = {0022-3093},
year = {2024},
date = {2024-02-17},
urldate = {2024-01-01},
journal = {Journal of Non-Crystalline Solids},
volume = {629},
pages = {122870},
abstract = {Artificial Neural Networks (ANN) are powerful machine learning algorithms. In the context of glass science, ANNs have been successfully used to induce composition-property regression models. These models are already influencing the way that we design new glasses, moving away from the traditional “cook and look” approach to computer-aided design. In this work, we induced an ANN model for the glass transition temperature Tg for glasses of the family xM2O⋅(100−x)B2O3, where M = Li, Na, K, Rb, and Cs, using simple radial basis function neural networks (RBF) with only two neurons. Statistically, the results of our methodologies are comparable to other complex models (empirical or physical) available in the literature with more neurons, such as the recent GlassNet and the topological model proposed by Mauro and co-authors (2009). This result supports the idea that a simpler specialized model can perform better than a complex general model for a restricted training domain.},
keywords = {Artificial neural network, Glass transition temperature, Radial basis function, Simple neural network},
pubstate = {published},
tppubtype = {article}
}
2023
@article{Cassar_2023,
title = {GlassNet: A multitask deep neural network for predicting many glass properties},
author = {Daniel R. Cassar},
url = {https://doi.org/10.1016%2Fj.ceramint.2023.08.281},
doi = {10.1016/j.ceramint.2023.08.281},
year = {2023},
date = {2023-08-01},
urldate = {2023-08-01},
journal = {Ceramics International},
publisher = {Elsevier BV},
keywords = {},
pubstate = {published},
tppubtype = {article}
}
@article{WELCH2023118994,
title = {Cracking the Kauzmann paradox},
author = {Rebecca S. Welch and Edgar D. Zanotto and Collin J. Wilkinson and Daniel R. Cassar and Maziar Montazerian and John C. Mauro},
url = {https://www.sciencedirect.com/science/article/pii/S1359645423003257},
doi = {https://doi.org/10.1016/j.actamat.2023.118994},
issn = {1359-6454},
year = {2023},
date = {2023-01-01},
journal = {Acta Materialia},
volume = {254},
pages = {118994},
abstract = {The Kauzmann paradox and associated Kauzmann temperature are two of the most widely debated topics in glass science over the past eighty years. Both conceptualized by Walter Kauzmann in 1948, the Kauzmann paradox occurs when some supercooled liquids apparently exhibit a negative excess entropy at temperatures above absolute zero. The Kauzmann temperature is the temperature at which the excess entropy vanishes. This review provides a retrospective on the origin of these hypotheses and their study through energy landscapes, crystallization behavior, and viscosity models. We also provide a critical analysis of each approach. After nearly eighty years of research, there is no conclusive evidence that supports the concepts proposed by Kauzmann. As such, it can be concluded that future work should be focused elsewhere.},
keywords = {},
pubstate = {published},
tppubtype = {article}
}
@article{MANNAN2023119046,
title = {Glass hardness: Predicting composition and load effects via symbolic reasoning-informed machine learning},
author = {Sajid Mannan and Mohd Zaki and Suresh Bishnoi and Daniel R. Cassar and Jeanini Jiusti and Julio Cesar Ferreira Faria and Johan F. S. Christensen and Nitya Nand Gosvami and Morten M. Smedskjaer and Edgar Dutra Zanotto and N. M. Anoop Krishnan},
url = {https://www.sciencedirect.com/science/article/pii/S1359645423003774},
doi = {https://doi.org/10.1016/j.actamat.2023.119046},
issn = {1359-6454},
year = {2023},
date = {2023-01-01},
journal = {Acta Materialia},
volume = {255},
pages = {119046},
abstract = {Glass hardness varies in a non-linear fashion with the chemical composition and applied load, a phenomenon known as the indentation size effect (ISE), which is challenging to predict quantitatively. Here, using a curated dataset of over 3,000 inorganic glasses from the literature comprising the composition, indentation load, and hardness, we develop machine learning (ML) models to predict the composition and load dependence of Vickers hardness. Interestingly, when tested on new glass compositions unseen during the training, the standard data-driven ML model failed to capture the ISE. To address this gap, we combined an empirical expression (Bernhardt's equation) to describe the ISE with ML to develop a framework that incorporates the symbolic equation representing the domain reasoning in ML, namely Symbolic Reasoning-Informed ML Procedure (SRIMP). We show that the resulting SRIMP outperforms the data-driven ML model in predicting the ISE. Finally, we interpret the SRIMP model to understand the contribution of the glass network formers and modifiers toward composition and load-dependent (ISE) and load-independent hardness. The deconvolution of the hardness into load-dependent and load-independent terms paves the way toward a holistic understanding of the composition effect and ISE in glasses, enabling efficient and accelerated discovery of new glass compositions with targeted hardness.},
keywords = {Glass composition, Hardness, Indentation load, Indentation Size Effect (ISE), Machine Learning (ML)},
pubstate = {published},
tppubtype = {article}
}
Destaques de Daniel Cassar
Orientados e Supervisionados por Daniel Cassar

Sarah Peixoto Rodrigues Freire
Vínculo: Iniciação Científica
Instituição: Centro Nacional de Pesquisa em Energia e Materiais (CNPEM)
Laboratório: Ilum – Escola de Ciência
Projeto: Projeto inverso de novos vidros bioativos. (CNPq)